The Boundaries of Meaningful Inquiry
1. Abstract
This paper argues that knowledge is intrinsically linked to prediction. We define the essential criteria for any knowledge system to facilitate prediction: time, space, causality, and discrete information. These criteria are fundamental necessities rather than mere practical tools. Our framework extends beyond human epistemology, proposing a universal theory applicable to all possible knowledge systems. We contend that discussing universes without predictive capabilities is inherently meaningless, providing profound insights into the nature of reality.
2. Introduction
2.1 Background and Motivation
We aim to establish prediction as the cornerstone of knowledge, proposing a unified theory that transcends traditional epistemological frameworks. We examine the necessary conditions that enable prediction, and discuss the broader implications for our understanding of reality. Additionally, we address how this framework resolves longstanding philosophical dilemmas and engages with contemporary scientific theories, such as quantum mechanics, to demonstrate its robustness and relevance.
Prediction plays a vital role across diverse fields, from science to daily life. This paper shows how prediction forms the basis of knowledge by demonstrating its central role in understanding reality.
2.2 Addressing Hume's Problem of Induction
Consider Hume's Problem of Induction—how we can justify our belief that the future will resemble the past. Hume argued that we can have no logical justification for assuming that patterns observed in the past will continue into the future, seemingly undermining the foundation of all scientific reasoning. However, our framework reveals that the consistency of past and future isn't something we need to justify through reason or experience, but rather a logical prerequisite for any predictive system to exist at all. Just as a self cannot exist without the ability to refer to itself across time, prediction—and thus knowledge—cannot exist without some degree of consistency between past and future states.
When we understand that knowledge is inseparable from prediction, we see that Hume's problem dissolves: we don't need to justify the assumption that patterns persist—rather, the very existence of predictive systems (like consciousness) proves that patterns must persist enough for prediction to be possible. The real question isn't how we can know the future will resemble the past, but rather how much consistency is necessary to maintain predictive systems.
3. Historical Background
3.1 Kant's Transcendental Approach
Kant's transcendental philosophy aimed to uncover the conditions of possibility for experience and knowledge in a rational agent. He argued that certain a priori structures must be in place to make coherent understanding possible.
3.1.1 Kant's Key Insights
- Pure Forms of Intuition: Space and time as a priori frameworks that necessarily structure sensible intuition, enabling synthetic a priori knowledge.
- Categories of Understanding: Pure concepts (including causality and substance) that organize experience into coherent knowledge by providing the rules for synthesis.
3.1.2 Limitations of Kant's Approach
- Complex Structure: While establishing space, time, and causality as necessary for experience, Kant's system required separate transcendental deductions for each element.
- Limited Scope: Kant's transcendental deductions are grounded in the specific structure of cognition, limiting its applicability to other potential knowledge systems.
3.2 Advancement Through the Predictive Framework
Our framework builds upon Kant's insights while identifying prediction as the more fundamental basis:
3.2.1 Distinctive Features
- Simpler Foundation: Grounds necessary conditions in prediction itself, providing a simpler explanation – in line with Occam's Razor – from which space, time, causality, and other conditions follow.
- Broader Scope: Extends to any system capable of prediction, deriving the same key features Kant identified (space, time, causality) from these more fundamental predictive requirements.
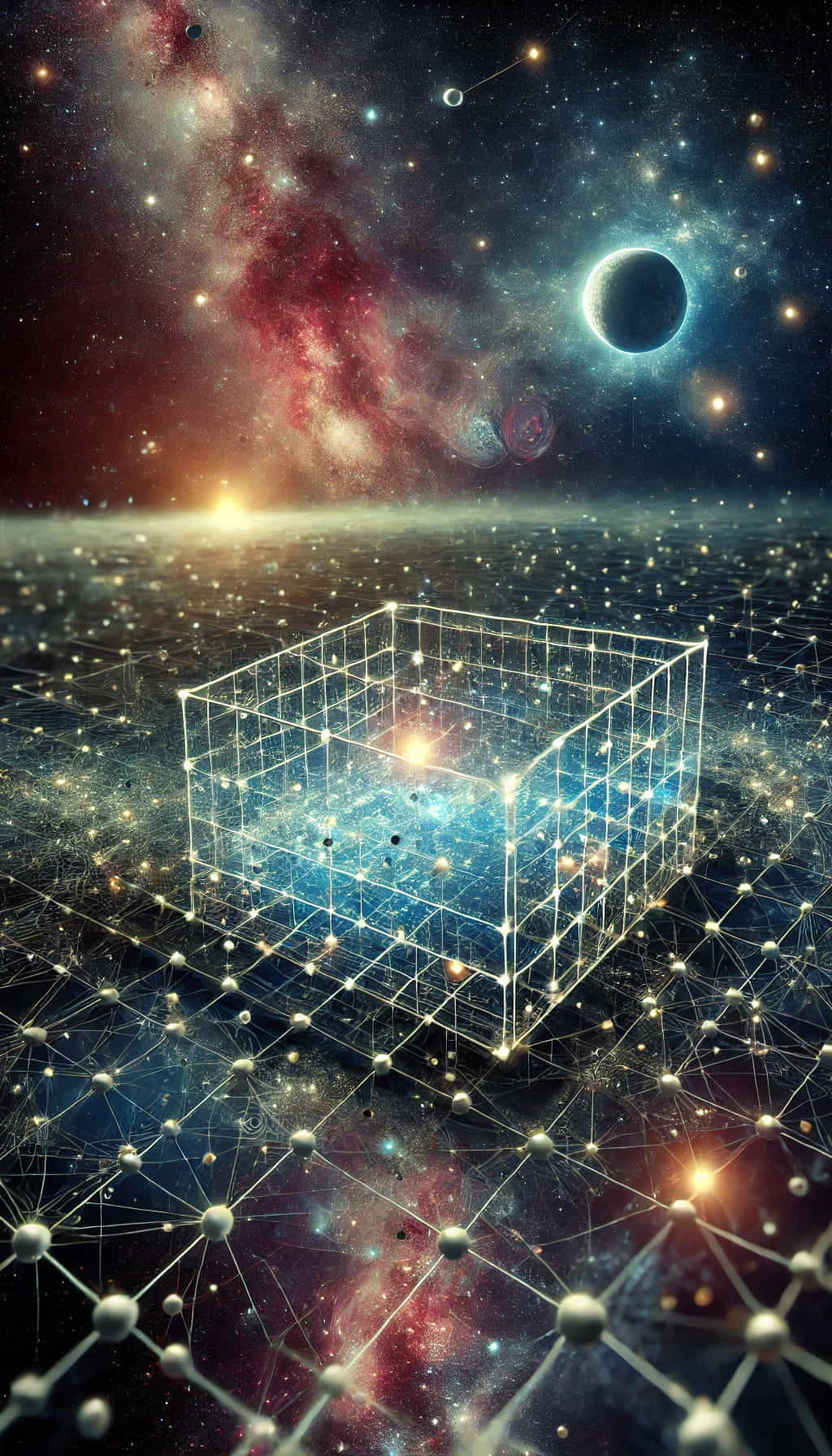
Universe 00110000
4. Core Argument Structure
4.1 Prediction as the Foundation of Knowledge
Knowledge inherently involves prediction. To know something is to anticipate its future behavior based on current and past information. This framework contends that all forms of knowledge are fundamentally predictive, with several key implications:
- Knowledge Systems Require Prediction: Systems incapable of prediction cannot possess coherent knowledge, as understanding inherently involves anticipating behavior.
- Scope of Knowledge: The boundaries of what can be known are defined by predictive capabilities. Understanding extends only as far as our ability to make reliable predictions.
- Universal Framework: This establishes universal laws governing all knowledge systems, transcending specific implementations.
4.1.1 Object Identity and Predictive Consistency
Object identity itself emerges from predictive consistency. This insight provides a novel resolution to the classical Ship of Theseus paradox: if every plank and component of a ship is gradually replaced, is it still the same ship? And if the original parts were used to build another ship, which is the "true" Ship of Theseus?
Through our predictive framework, this paradox dissolves. An object's identity is not determined by material continuity but by predictive consistency. The ship remains "the same" as long as its behavior and properties remain predictable within our established framework. This explains why we intuitively consider a renovated ship the "same" vessel—it maintains consistent predictive relationships with its environment and our expectations, regardless of material replacement.
This understanding of identity through prediction extends beyond physical objects to all forms of knowledge. Even abstract concepts maintain their identity through predictive consistency rather than any underlying substance or essence.
4.1.2 Historical Knowledge, Cogito and Mathematical Truths
Historical knowledge, even when focused on seemingly static facts, implies an assumption about the stability and consistency of human memory, records, and interpretive frameworks. Knowledge of the past is never purely retrospective—it always involves forward-looking elements about how this knowledge will hold up to future scrutiny, new evidence, and continued application.
Certain knowledge (like cogito ergo sum) is actually predictive—a self cannot exist without the ability to refer to itself across time, just as prediction cannot exist without defined relationships between past, present, and future. When we think, we are predicting our own continuity: the self must persist to complete the thought, thinking itself occurs over time.
This extends even to mathematical truths—when we claim to know that 2+2=4, we are making a prediction that this relationship will hold true not only in the present moment of our understanding but in all future calculations. We are predicting that no contradiction will emerge that would invalidate this truth.
4.2 Requirements
4.2.1 Time Sequence and Directionality
4.2.1.1 Basic Temporal Framework
A temporal structure is essential for anticipating future events based on past and present information, enabling the modeling of cause-and-effect relationships.
4.2.1.2 Arrow of Time as Logical Necessity
The unidirectional flow of time is essential for maintaining predictive validity. Reversing time would invalidate all predictive knowledge, making the forward direction of time a logical necessity.
- Time must progress forward to sustain predictive accuracy.
- The arrow of time is dictated by the requirements of prediction.
4.2.1.3 Logical Proof of Time Sequence
- Assume time can reverse:
- Past becomes future and vice versa.
- Predictive knowledge becomes invalid.
- The existence of predictors is contradicted.
- Conclusion:
- Time must move forward.
- This is a logical necessity, not just a physical law.
- The arrow of time is inherently linked to prediction and thus knowledge.
4.2.1.4 Implications for Predictive Systems
- Information Flow: Must move from past to future.
- Knowledge Accumulation: Requires stable temporal progression.
4.2.2 Space
Space emerges as a logical necessity even when considering the simplest possible predictor—one that only predicts its own continued existence.
4.2.2.1 Existence Location
Consider a minimal predictor that only maintains one prediction: "I will continue to exist." Even this most basic predictor must exist somewhere rather than nowhere.
4.2.2.2 Knowledge Storage
Even our minimal predictor requires:
- A place to exist
- A place to store its single prediction
- A place to store the verification of that prediction
These requirements cannot be avoided by making the predictor simpler - they represent the absolute minimum needed for any prediction to occur. While the predictor's "knowledge" might be as simple as a single bit, that bit must exist somewhere.
4.2.3 Causal Relationships
Causality emerges as a logical necessity for any predictive system. Without consistent patterns of influence between states or events, prediction becomes impossible by definition. This necessity transcends specific physical implementations of causal mechanisms.
4.2.3.1 Logical Necessity for Prediction
For prediction to be possible, there must exist reliable relationships between states that allow future conditions to be anticipated based on present information. This requirement is not merely practical but logically necessary - in a system without causal relationships, no basis for prediction could exist.
- Definition: Consistent patterns of influence between states or events that enable prediction
- Core Requirements:
- Stable influence patterns that persist across time
- Consistent relationships between causes and effects
- Clear temporal ordering of related events
4.2.3.2 Integration with Quantum Mechanics
While quantum mechanics challenges classical deterministic causation, it maintains probabilistic causal relationships that enable prediction. The quantum measurement problem demonstrates how even fundamentally probabilistic systems require stable causal structures to support prediction.
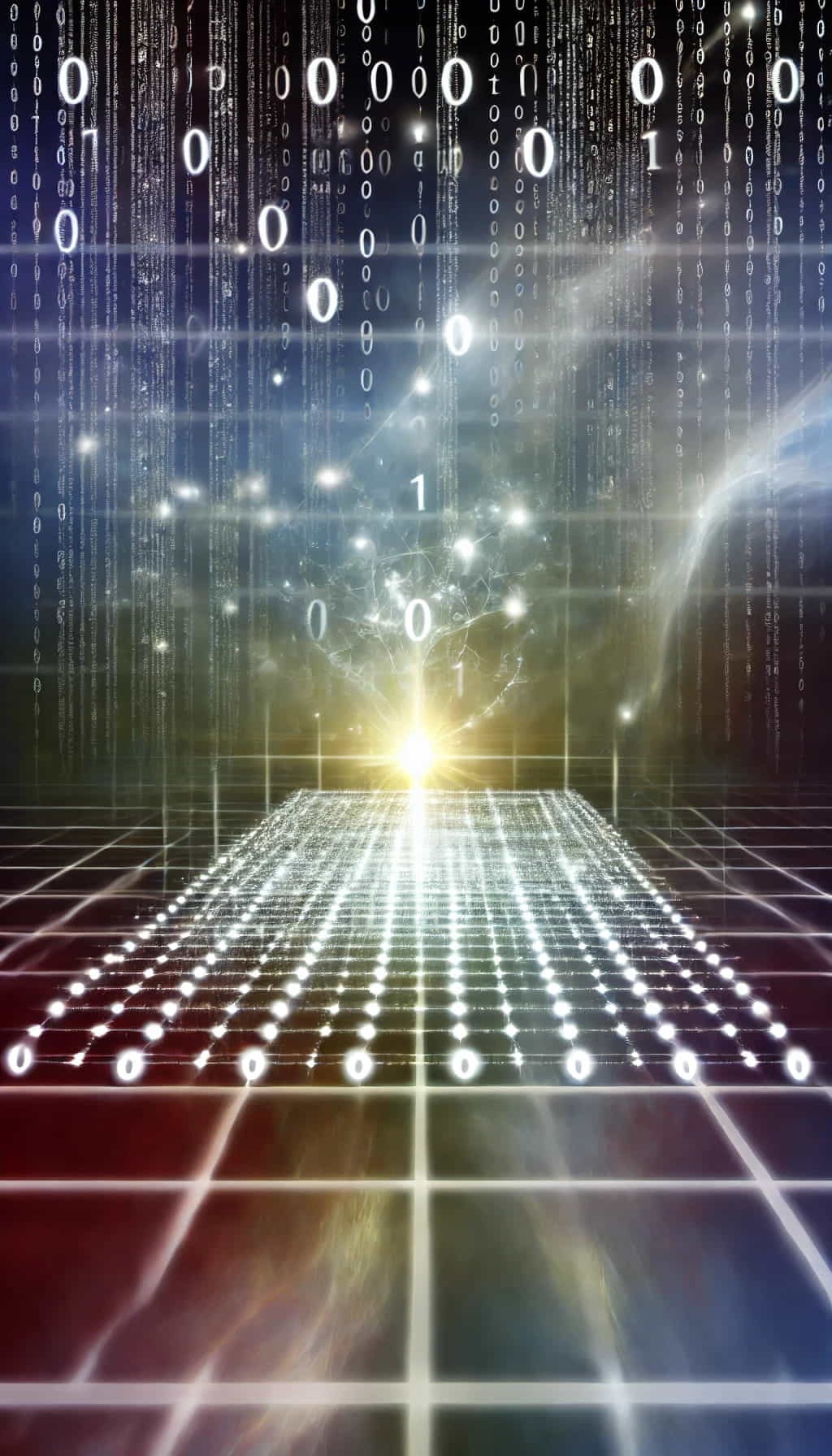
Universe 00110000
4.2.4 Discrete Information
4.2.4.1 Definition
The discreteness of information proves particularly crucial—the inherent binary split between predictor and predicted isn't merely a convenient way of thinking about prediction; it represents a logical necessity without which prediction itself is impossible. For a prediction to be meaningful, there must be a clear separation between the entity making the prediction (the subject, the predictor) and the event or state being predicted (the object, the predicted). This fundamental binary distinction forms the bedrock upon which all complex structures of knowledge are built.
4.2.4.2 Logical Necessity of Binary Separation
The elegant simplicity of binary logic (true/false, 1/0) is not an arbitrary system imposed upon reality, but rather the inevitable consequence of the predictor-predicted relationship that makes knowledge possible. We need not posit a separate domain of truth or grapple with how abstract objects relate to concrete reality—binary logic arises naturally and necessarily from the foundational requirements of prediction itself.
4.2.4.3 The Basis of Discreteness
The basis of discreteness is the separation between the predictor and predicted, even if the predictor attempts to predict its own future states. This separation establishing a foundational discreteness where the predicted works as an irreducible unit and the distinction is clear-cut and binary.
4.2.4.4 Implications for Knowledge Systems
- Clear Distinction: Ensures that predictors and predicted entities remain distinct, preventing logical ambiguities.
- Irreducible Units: The predicted acts as an indivisible unit, simplifying the modeling and understanding of complex systems.
- Binary Clarity: Maintains a straightforward binary framework that supports reliable and consistent predictions.
- Foundation for Complex Structures: Provides a stable base upon which more intricate knowledge systems can be constructed.
4.2.4.5 Addressing Self-Prediction Challenges
Even when predictors attempt to predict their own future states, the discrete separation remains intact. Perfect prediction remains impossible due to the infinite regress that arises whenever a prediction must include a prediction about the predictor itself. This fundamental limitation, rather than compromising the predictive framework, actually ensures its integrity by maintaining consistent boundaries and enabling reliable knowledge acquisition within those bounds.
4.3 Impossibility Arguments
4.3.1 Inability to Discuss Universes Without Predictors
Without predictive capabilities, properties or phenomena lack a framework for understanding, since to know and understand anything we must be able to make predictions about it. Therefore, discussing universes that do not contain knowledge systems - which necessarily means they lack predictors - is inherently meaningless. Comprehension and inquiry depend on the ability to predict outcomes. Without prediction, understanding and investigation lose coherence.
5. Implications for Knowledge and Existence
5.1 The Nature of Understanding
- Predictive Foundation: Understanding involves anticipating behavior based on information.
- Knowledge Process: Accumulates and applies information for forecasting.
- Theoretical Implications:
- Provides a unified approach to knowledge across disciplines.
- Establishes clear criteria for evaluating theories.
6. Quantum Mechanics Integration
6.1 Quantum Indeterminacy Within the Framework
Quantum mechanics introduces elements of indeterminacy that challenge deterministic predictive models. However, probabilistic prediction remains integral to this framework. By accommodating statistical predictions, the framework addresses quantum indeterminacy, allowing for both deterministic and probabilistic predictive mechanisms.
6.2 Probabilistic vs. Deterministic Prediction
The framework differentiates between deterministic and probabilistic prediction, asserting that both are essential for comprehensive predictive systems. Deterministic prediction relies on precise cause-and-effect relationships, while probabilistic prediction handles statistical likelihoods.
6.3 Quantum Measurement Problem Details
6.3.1 Traditional Problem Analysis
The quantum measurement problem questions how measurement collapses the wave function and what constitutes a measurement. These questions significantly impact our understanding of reality and the role of observers in quantum systems.
6.3.2 Framework Insights
- Measurement and Prediction: Measurement involves prediction by anticipating outcomes based on the wave function's probabilistic distribution.
- Discrete Information Requirement: The predictor-predicted relationship necessitates discrete information, potentially explaining the quantized nature of measurement outcomes.
- Wave Function Collapse: Can be seen as establishing a definitive predictor-predicted relationship, transitioning from superposition to a single outcome.
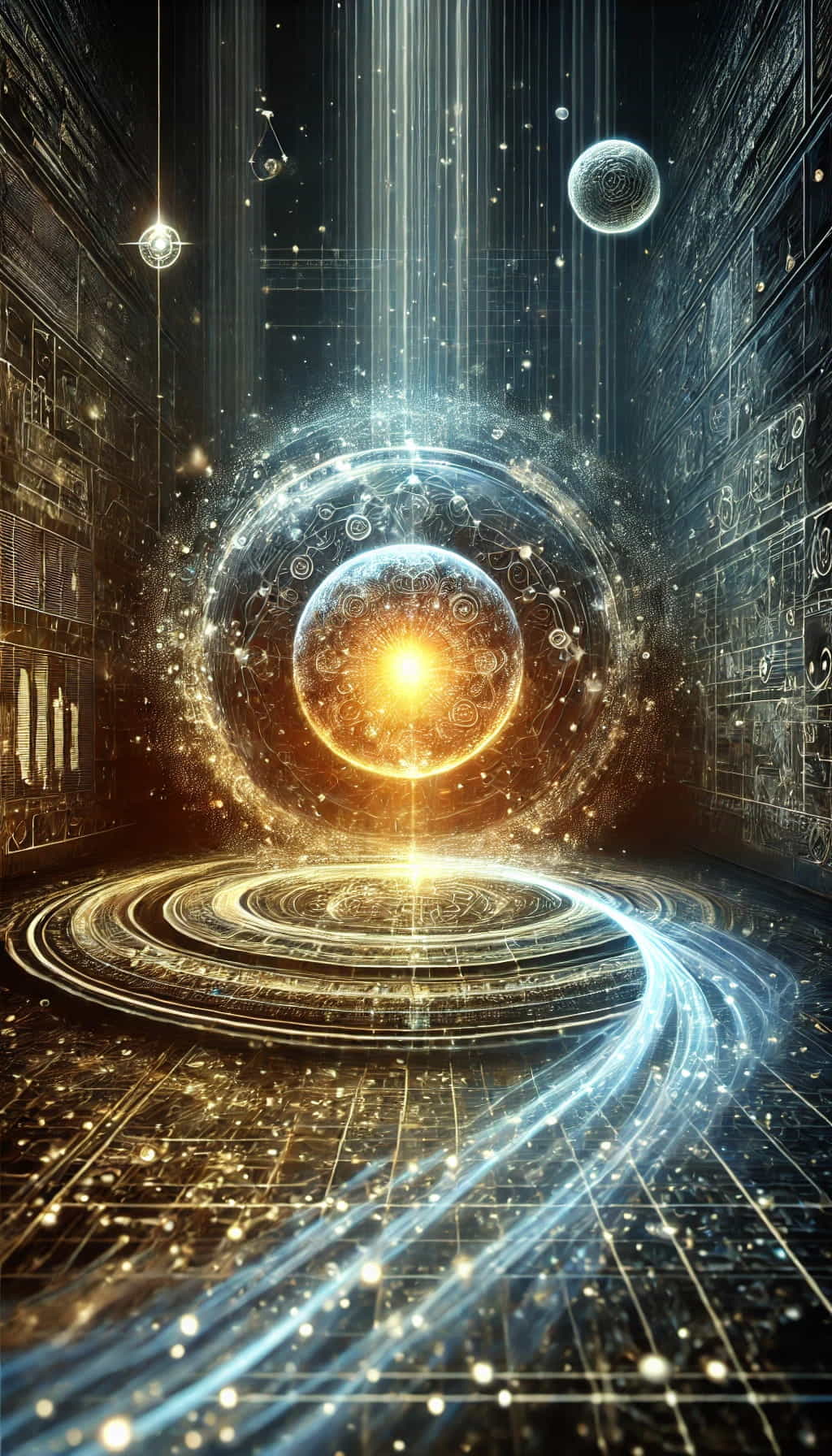
Universe 00110000
7. New Framework for Scientific Investigation
Our framework introduces a fundamental reorganization of scientific inquiry, establishing clear boundaries between different types of questions and their appropriate investigative approaches. This methodology not only resolves historical confusions but also provides a clear path forward for future research. By identifying logical necessities, we can more effectively direct scientific efforts and avoid pursuing logically impossible research directions.
7.1 Classification of Questions
The framework divides scientific inquiry into two distinct categories, each requiring different investigative approaches and carrying different implications for what can be known:
7.1.1 Logical Necessities
These represent the foundational requirements for any predictive system to exist. They are not empirically discovered but rather deduced from the requirements of prediction itself:
- Derived from predictor requirements
- Immutable while maintaining prediction
- Addressed through deductive analysis
- Examples: space, time, causality, discrete information
7.1.2 Physical Reality
These encompass the specific ways in which logical necessities are manifested in physical reality, allowing for variation while maintaining predictive capability:
- Operate within logical constraints
- Can vary while maintaining prediction
- Require empirical investigation
- Examples: physical constants, force strengths, particle properties
7.1.3 Research Methodology
This classification leads to a structured approach for conducting research, enabling more efficient and focused scientific investigation:
7.1.3.1 Investigation Process
The framework provides a systematic approach to categorizing and addressing scientific questions:
- Classify question type (logical vs. physical)
- Identify fundamental predictive requirements
- Determine appropriate investigative approach
- Set clear boundaries for empirical research
7.2 Historical Confusions and Resolution
Understanding this framework helps resolve numerous historical confusions in scientific inquiry, where logical necessities were often mistaken for physical contingencies:
7.2.1 Misclassified Questions
Fundamental questions in physics have been approached from the wrong perspective, treating logical necessities as physical phenomena:
- Arrow of time treated as physical rather than logical
- Quantum measurement problem approached as physical mechanism
7.2.2 Common Historical Errors
These misclassifications led to several persistent errors in scientific investigation:
- Seeking physical explanations for logical necessities
- Attempting to vary logically immutable features
- Failing to distinguish between necessary and contingent aspects
8. The Fundamental Importance of the Predictive Framework
Scientific achievements range from practical discoveries to natural laws to unifying theories. This framework surpasses these categories by explaining why reality must possess its most fundamental features. While other scientific works describe physical mechanisms within our universe, this framework elucidates the logical necessities for any universe containing knowledge.
8.1 Unique Explanatory Power
This framework fundamentally explains:
- The necessity of time's arrow
- The existence of causality
- The discreteness of information
- The requirement for spatial distinction
- The nature of quantum measurement
8.2 Universal Implications
The framework's universal applicability is key to its importance:
- Applicable to any universe containing knowledge.
- Sets absolute boundaries on what can exist in any reality that supports knowledge.
- Explains why scientific investigation itself is possible.
9. Conclusion
This framework represents the deepest level of scientific explanation by demonstrating not just how things work, but why reality must have the basic features that allow things to work. It provides the foundational basis upon which all other scientific understanding rests, making it uniquely important among scientific theories.
Its meta-scientific position, combined with its explanatory power for fundamental aspects of reality, establishes this framework as one of the most significant contributions to scientific understanding. It moves beyond merely describing the universe to explaining why it must possess its basic features, representing a fundamental advance in human knowledge.