Emotional Pattern Analytics: A New Frontier in Understanding Collective Well-being
Overview
Emotional Pattern Analytics is a groundbreaking interdisciplinary field that focuses on understanding, modeling, and predicting the collective emotional states of populations on a large scale. It combines principles and techniques from various disciplines, including psychology, sociology, data science, complex systems theory, and computational social science.
How Emotional Pattern Analytics Works
Emotional Pattern Analytics treats collective emotions as a complex system that can be analyzed and predicted using advanced computational methods. It recognizes that emotional states are influenced by a wide range of factors, such as established norms, economic conditions, environmental changes, social interactions, and media exposure.
By studying these factors and their interactions, Emotional Pattern Analytics aims to identify regularities that enable the forecasting of collective emotional states, much like predicting weather patterns. This methodology utilizes large datasets gathered from various sources, including social media and news articles, to gain comprehensive insights into collective emotional expressions.
Historical analysis plays a crucial role in identifying patterns and trends in emotional states over time. By examining past emotional data, researchers can uncover recurring cycles and seasonal variations, which are essential for accurate forecasting.
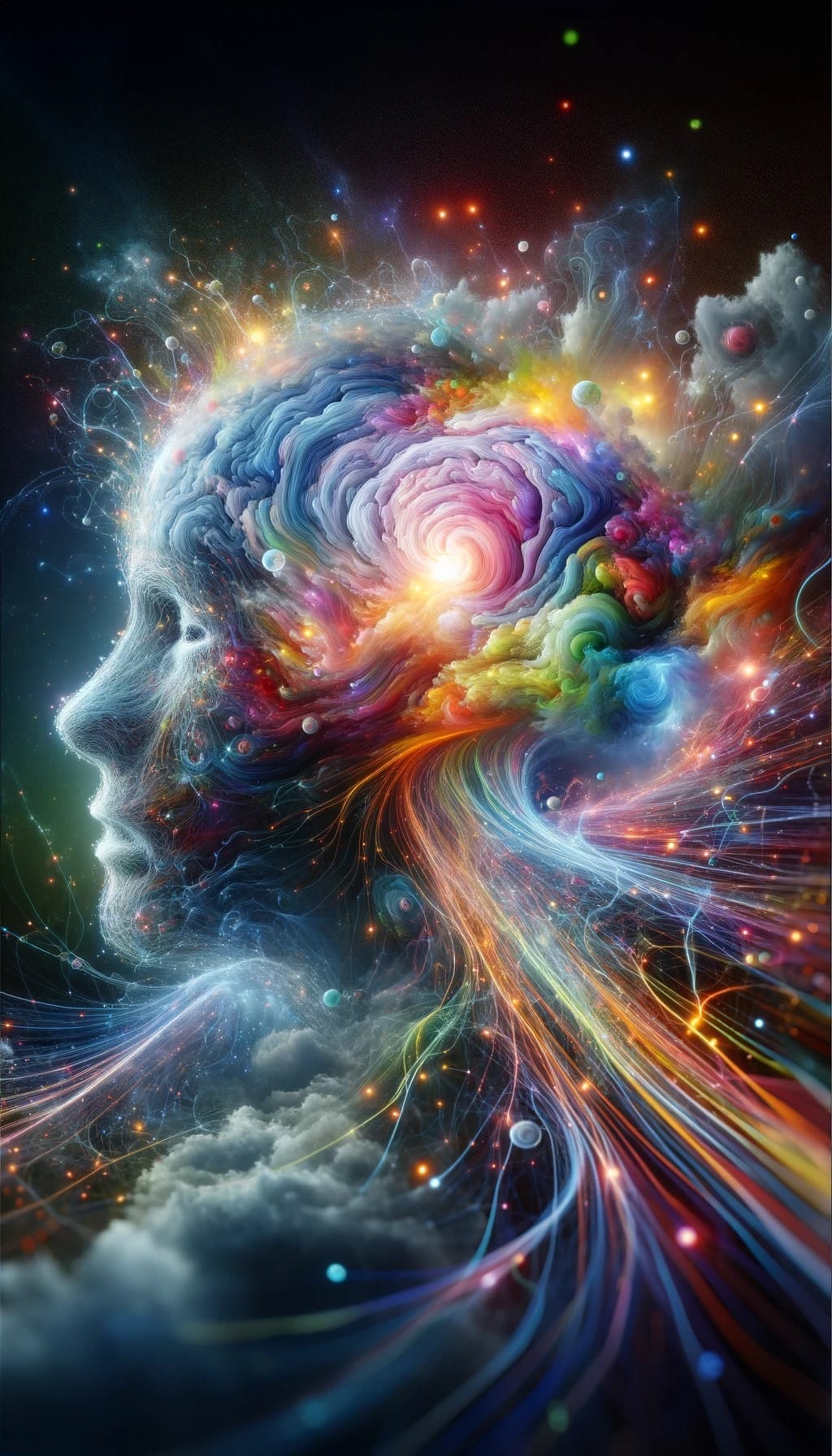
Universe 00110000
Key Techniques in Emotional Pattern Analytics
- Sentiment Analysis and Natural Language Processing (NLP): NLP techniques, such as sentiment analysis and emotion classification, are used to extract emotional information from text data. Machine learning algorithms are trained to assign sentiment scores and categorize emotions based on the linguistic features of the text.
- Time Series Analysis: Emotional data is analyzed as time series to identify patterns, trends, and seasonality. Techniques such as autoregressive integrated moving average (ARIMA) and long short-term memory (LSTM) neural networks are employed to model and forecast emotional trajectories.
- Geospatial Analysis: Emotional data is mapped and analyzed in a geographic context to understand the spatial distribution and clustering of emotions. Techniques such as spatial autocorrelation and geo-visualization are used to identify emotional hotspots and patterns of emotional contagion.
- Network Analysis: Social network analysis techniques are applied to understand the propagation of emotions through social connections. Metrics such as centrality, clustering, and community detection are used to identify influential individuals and groups in the spread of emotional states.
- Advanced Predictive Techniques: Techniques like deep learning are employed to improve the accuracy of sentiment analysis and emotional forecasting models. These methods allow for more sophisticated and precise predictions.
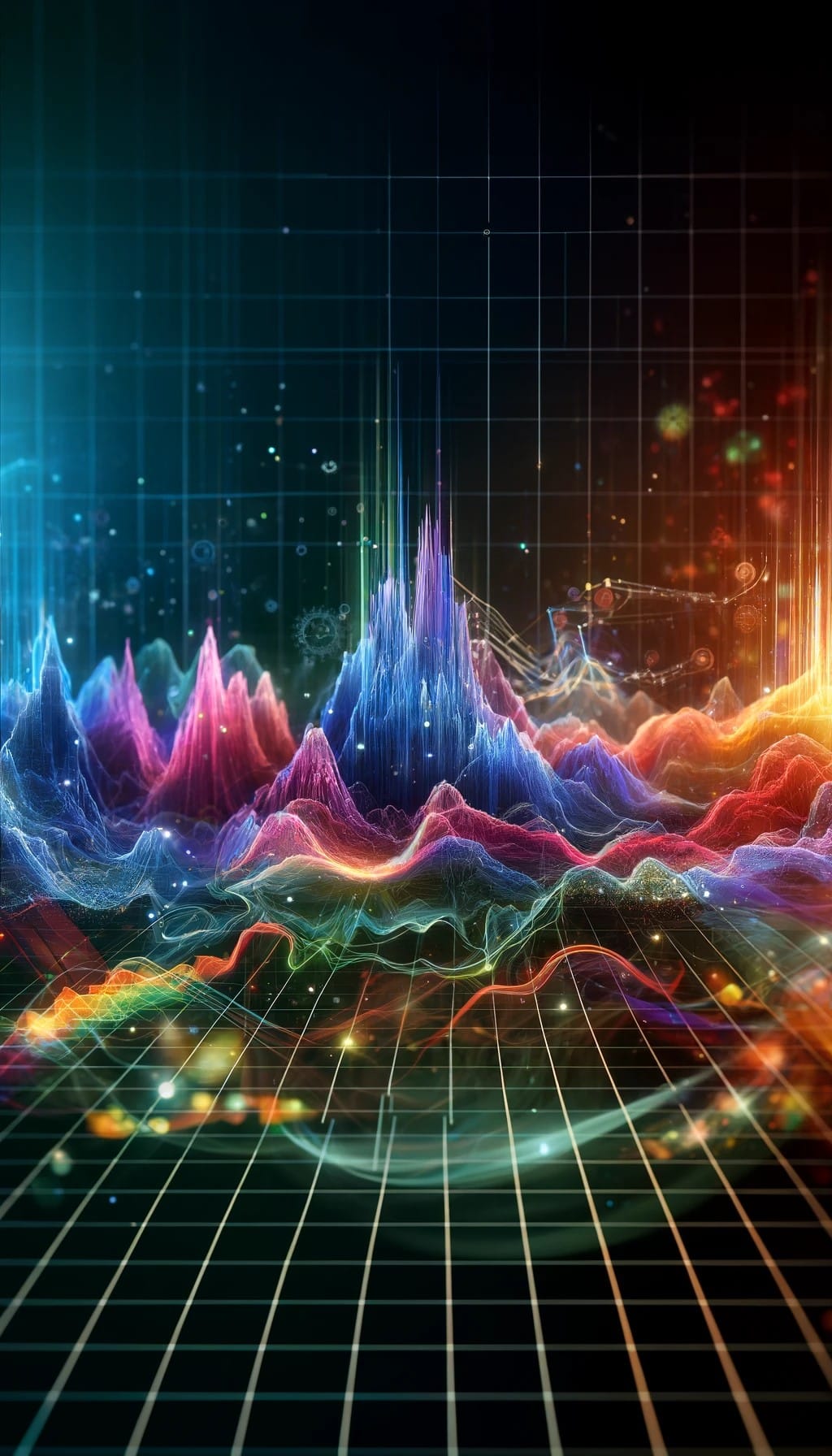
Universe 00110000
Developing a Mathematical Framework
Emotional Pattern Analytics aims to develop a rigorous mathematical framework to capture the complex dynamics of societal well-being. This framework integrates concepts from stochastic processes, dynamical systems theory, and network theory to model the intricate interactions and emergent properties of emotional contagion in large-scale social networks.
By providing a quantitative foundation for understanding and predicting collective emotional states, Emotional Pattern Analytics offers a novel approach to promoting emotional resilience and informed decision-making in various domains.
Conclusion
Emotional Pattern Analytics represents a promising frontier in understanding and predicting collective emotional states on a global scale. By leveraging large datasets, advanced computational techniques, and insights from various disciplines, this field offers a groundbreaking framework for analyzing the complex dynamics of societal well-being.
As research in Emotional Pattern Analytics progresses, it has the potential to revolutionize our approach to promoting emotional resilience and building more resilient communities in the face of various challenges.